This article would be a useful resource for companies dealing with any type of coverage and want to make their business even better with the latest technologies. You will find out what market challenges industry players face, how ML implementations improve a variety of business processes, and how market leaders benefit from them now.
Main Market Challenges
According to a 2020 PwC survey, financial CEOs consider over-regulation to be the biggest threat to business growth and worry about the economic environment the least. The survey results also showed that most insurance companies realize the challenges coming for the next 12 months and stick to no-risk strategies to survive market turbulence. Market leaders prefer to use these challenges to their advantage by enhancing services with digital transformations.
Even at times of uncertainty, there’s a fierce competition among insurers, which still doesn’t stop new industry players from starting their business in this niche. Many of them rely on improving client service to stand out from other market players. Considering the mentioned challenges, coverage businesses must be ready to adapt to changes and unforeseen situations at all levels of the organization to stay profitable and competitive. Machine learning is one of the keys to success.
However, the recent Accenture report says that only a small percent of industry players are ready to adopt AI solutions. Meanwhile, almost 80% are convinced that the future of financial business is impossible without human-machine collaboration.
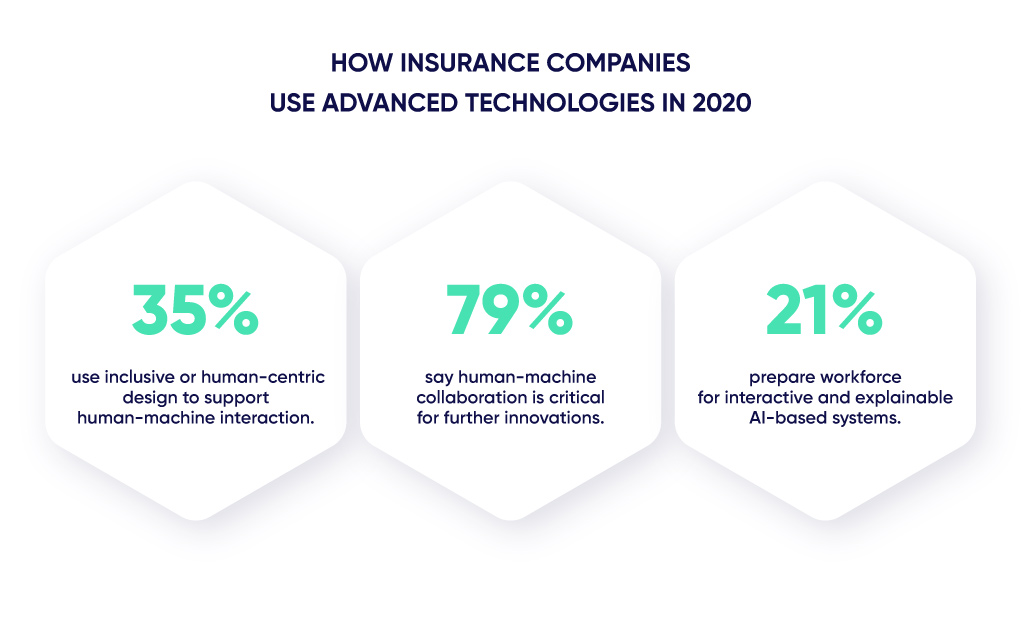
While insurance industry leaders already use the benefits of machine learning and artificial intelligence, their competitors have yet to struggle over gaining higher productivity and profitability. They must analyze the available resources for the implementation of advanced technologies or engage data science partners to get the necessary help.
How ML Improves Insurance Business
Any insurance company realizes the opportunities provided by modern technologies in operational efficiency, cost reduction, fraud detection and prevention. For this reason, they increase budgets for the implementation of ML models. The most demanded techniques related to machine learning in this field are:
- Predictive analytics;
- Image recognition;
- Text analysis (chatbots and voice assistants);
- Claim anomalies detection.
The table below describes the main areas that machine learning enhances efficiently, and the key results obtained after its implementation.
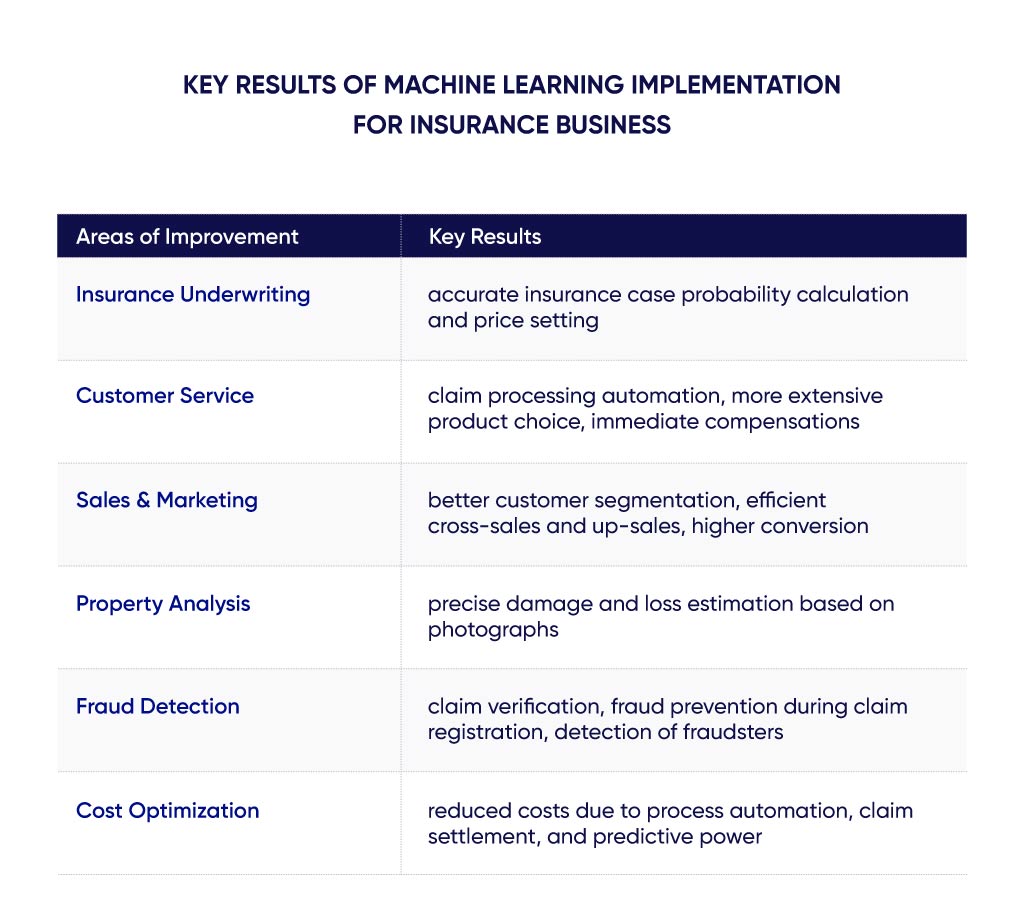
Insurance Underwriting
When setting prices for contracts and services, insurers rely on the underwriting process, i. e. the calculation of the accident case probability and potential risk assessment for an individual client. Agencies set a specific price by analyzing data on the policyholder, the object to cover, statistics of incident occurrence in similar situations, etc. After data analysis, insurers can accurately define the insurance pricing for each client by using ML models.
As a case in point, Allianz SE, one of the leading financial corporations in the world, uses machine learning to generate voluntary health insurance quotes of new corporate clients. One more advantage for the company was obtaining even more informative big data collected after the interpretation of the ML model results.
Customer Service
Smart technologies automate client interaction and significantly increase its quality from the first claim registration to deal closing. Besides delegating the initial communication with clients to chatbots, insurers can create more personalized and fair coverage plans. Their clients will be able to get a guaranteed payment in case of loss or damage in the shortest terms. With the integration of IoT systems at offices, it’s possible to enhance customer experience on the premises by applying ML methods based on the sensor-generated data. Business owners can even get furniture organized in the most convenient way to provide services faster for a larger number of people.
Property Analysis
Insurers can estimate and prioritize claims more efficiently with machine learning, computer vision, and image recognition technologies. It is especially relevant when an insurance agent is on the stage of damage or loss estimation and needs an accurate result. By using ML models that recognize damaged elements from photographs, the price for their replacement or repair is defined automatically.
Sales & Marketing
Financial services don’t use machine learning only for the back-office functions anymore. The high-quality models ensure sales growth and better customer satisfaction. Thus, your sales and marketing efforts can be enhanced by:
- Improved segmentation. Businesses can segment customers according to various criteria. For instance, by social and age factors, the information on used insurance products, their interaction with the support service and other company’s departments, etc. All of this forms a multi-factor model with thousands of data that only an expert data scientist can enhance by using ML methods.
- More efficient cross-selling and up-selling. Machine learning models let companies increase profit by identifying people that are the most likely to purchase more products on the spot or after some time.
- Higher sales conversion rate. With predictive modeling, insurers will be able to identify loyal and disloyal people to a particular policy type. Thus, they increase the sales conversion rate by offering the right policies to the right buyers without wasting time on those who aren't interested in particular services.
Fraud Detection
According to ACFE Insurance Fraud Book, the money loss from fraud is $80 to $100 billion a year in the developed countries. For this reason, industry players use machine learning methods as a protective measure from criminals and a way to reduce money loss. These methods help insurers and police officers to reveal and investigate the following fraud types:
- Fraud by agents. As an example, some agents usually exaggerate facts about car damage to get a higher percentage from the compensation. When an insurance employee takes part in crime with service station workers and a customer, they can overstate the price for spare parts and car repair. In this case, partners in crime divide the difference between actual damage costs and compensation.
- Fraud by customers. People who want to get money from an insurer quickly can stage a car accident or theft. They may also buy life, health, or property coverage from different insurers to get compensation for one accident several times.
Here are the ways that insurance fraud detection using machine learning works to protect companies and save money:
- Claim verification. Smart technologies identify the same data in different insurance claims, detect unusual or suspicious connections between different customers, devices, policies, and application data. They also analyze historical data about the policyholder and the insured property;
- Fraud prevention during claim registration. In this case, companies can identify suspicious relationships between the existing and new clients, phone numbers, IP addresses, devices, bank accounts, repair shops, and medical providers.
- Detection of fraudsters. Advanced technologies let insurers get data on customers’ and agents’ behavior and compare it to the previously collected data on fraudulent behavior. ML methods help detect agents that work differently from others or notice a considerable change in their habitual behavior. Social media analysis reveals relationships between agents and customers and signs of their partnership in fraud.
Budget Optimization
There are three major factors that make ML solutions best at optimizing budget at financial coverage entities:
- Process Automation. By eliminating routine tasks, insurers reduce human resources and cut expenses on wages. Sometimes there’s a collaboration between a human and a machine. In this case, you can reduce operational costs by eliminating human-generated mistakes that might lead to loss of money.
- Claim settlement. Machine learning allows insurers to provide faster compensations. Sometimes it may cause overpay. This problem is especially relevant for insurers who faced the need to process a great number of claims simultaneously from people whose property was destroyed because of a natural disaster. It is possible to optimize the ceiling amount of immediate payments by analyzing the claim content and history.
- Predictive Power. In healthcare, predicting the cost of the insured person's treatment is a good way to reduce expenses. If you know that the client's treatment will require a serious amount of money, you can arrange their visits to more qualified doctors. You can offer them to take previous diagnostics or monitor how they follow doctors' recommendations to reduce the price for treatment.
Prominent Use Cases
Here are some examples of modern insurance leaders that optimize their business processes with machine learning:
Manulife. One of the biggest Canadian-based insurers Manulife uses advanced technologies to handle data of over 26 million clients from all around the world. In 2018, the company began the four-year cooperation with Waterloo Artificial Intelligence Institute under the research and implementation of predictive models for a disability claim, fraud detection, and natural language processing for bettering its customer service.
Ping An Property & Casualty Insurance. Ping An is a Chinese company using big data and image recognition technologies to identify hidden risks and improve loss assessment. Earlier, the damaged car owners could spend an indefinite amount of time completing claims. Machine learning helped Ping An to reduce that time to only three minutes.
Progressive. This US-based corporation specializes in vehicle insurance and implemented ML algorithms to predict car accident possibilities for potential customers. Snapshot is a mobile app and a plugin device for vehicles created by Progressive to gather driving data and use it for further predictive analysis. Based on the analytics, Progressive attracts and builds long-term relationships with reliable and careful drivers.
Cost of Implementation
One cannot be straightforward about the prices for ML implementations. It usually varies depending on the company’s goals with machine learning, the rates offered by data science specialists or agencies, the number of specialists in a team, and their location. Many companies outsource data science tasks to specialists in other countries, seeing it as a great opportunity to save financial resources and equipment compared to in-house development. You can discuss any project idea for the fintech sector by contacting data science specialists directly, get advice from the company you are interested in, and see if this cooperation fits your vision and budget.
Conclusion
The integration of machine learning into insurance business processes gives you a competitive advantage. By optimizing underwriting and fraud detection processes, risks can be assessed more accurately and huge financial losses avoided. More efficient customer experience and focus on improving marketing and sales efforts with ML help to retain clients and offer them exactly what they want. Thus, the company’s profits increase, and predictive powers provide valuable insight to keep those profits high. If you decided that now it’s time to improve your business, our team is ready to consult you on the specifics of machine learning implementation. Feel free to contact us and get high-quality ML solutions from our data scientists.